Combination therapies and predicting regimens
Quantitative systems pharmacology (QSP) modeling uses already available clinical trial data to evaluate novel therapeutic regimens prior to beginning new clinical trials, helping to accelerate drug development.
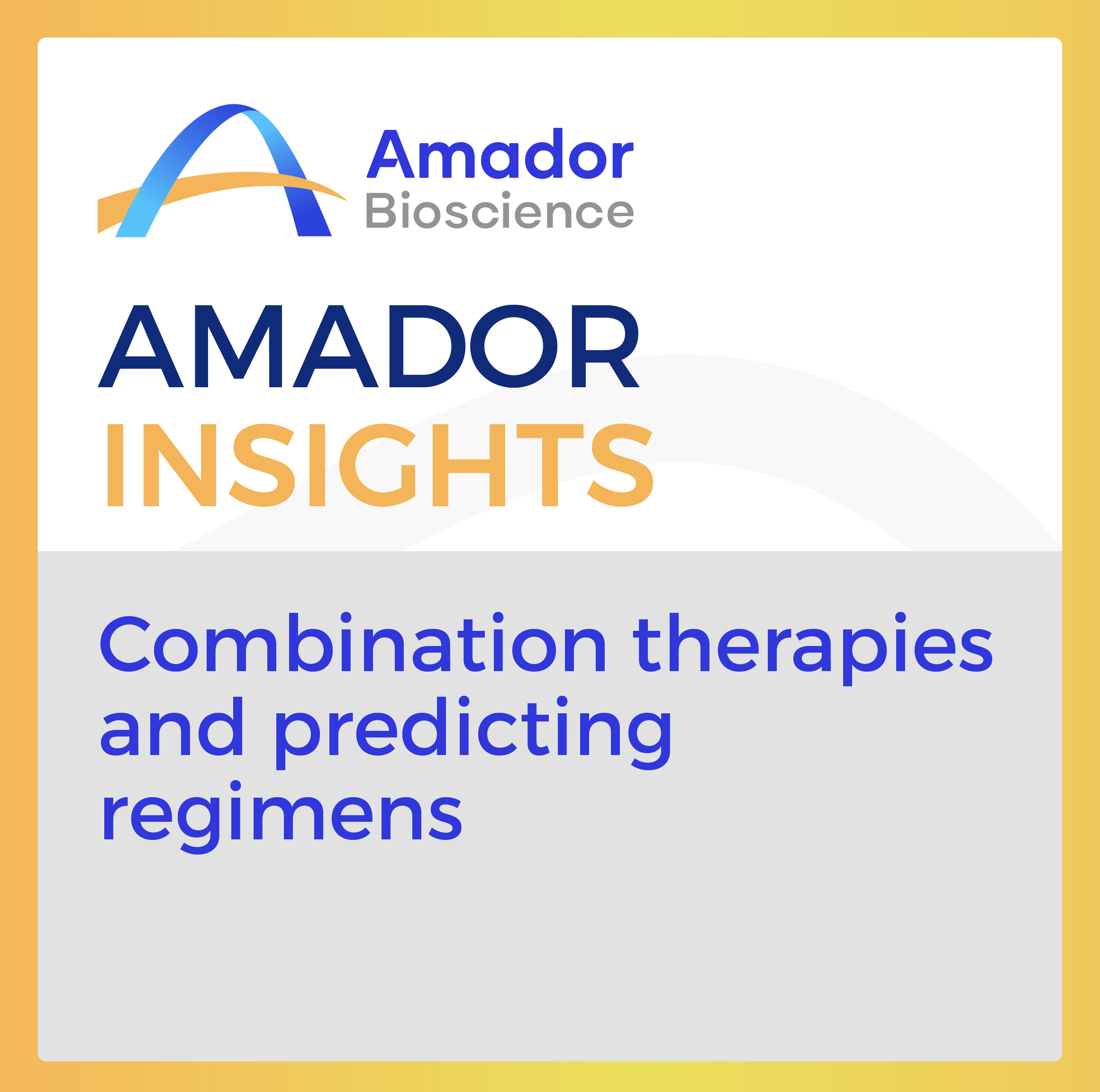
The first immune checkpoint inhibitor (ICI) was approved by the FDA in 2011 and since then this class has expanded to 10 distinct agents with 109 approvals. This makes the ICI class second only to kinase inhibitors in oncology approvals in 2000-2022[1]. The success of this class can be largely attributed to the flexibility of their indications. By targeting a patient’s immune response to a cancer rather than the cancer itself, the drugs are less reliant on specific mutations associated with different cancers. This flexibility does come with a tradeoff however: how does a sponsor decide what treatment regimen will best improve patient immune response to a specific cancer?
Amador CEO Bing Wang and CSO Lorin Roskos have previously explored the use of quantitative systems pharmacology (QSP) modeling for this very purpose in both melanoma[2] and metastatic breast cancer[3]. Using available clinical trial data on patient responses to CTLA-4, PD-1, and/or PD-L1 checkpoint inhibitors, they and their co-authors built QSP models for each cancer type to evaluate what therapeutic regimens and patient features resulted in positive patient responses.
Building an effective QSP model involves integration of cellular and molecular interactions relevant to the therapy in question, as well as any known pharmacokinetic (PK) and pharmacodynamic (PD) data. These interactions are transformed into a series of equations to represent the complex system at play, and the performance of the model validated to insure it returns results comparable to known data. Once validated, the model can be manipulated to input actual or simulated patient feature information and alter treatment regimens to provide predictions for patient groups or treatment regimens that have not previously been performed. Check out the papers in full at the links below.
Simulations do not replace the need for in-patient evaluations, but they can provide information to make clinical trials more efficient to the benefit of both patients and sponsors. Patients are enrolled in treatments that are more likely to be effective for them, and sponsors have increased confidence in a trial providing informative results.
[1] Scott, E.C., Baines, A.C., Gong, Y. et al. Trends in the approval of cancer therapies by the FDA in the twenty-first century. Nat Rev Drug Discov 22, 625–640 (2023). https://doi.org/10.1038/s41573-023-00723-4
[2] Milberg, O., Gong, C., Jafarnejad, M. et al. A QSP Model for Predicting Clinical Responses to Monotherapy, Combination and Sequential Therapy Following CTLA-4, PD-1, and PD-L1 Checkpoint Blockade. Sci Rep 9, 11286 (2019). https://doi.org/10.1038/s41598-019-47802-4
[3] Wang, H., Milberg, O., Bartelink, I.H. et al. In silico simulation of a clinical trial with anti-CTLA-4 and anti-PD-L1 immunotherapies in metastatic breast cancer using a systems pharmacology model. R. Soc. open sci. 6: 190366 (2019). http://dx.doi.org/10.1098/rsos.190366